The AI landscape is evolving at an unprecedented pace. New foundational models are emerging, techniques to improve the intelligence are coming to life (context length improvements, chain of thought etc), AI-powered products are being launched, and the innovation in this space is staggering. However, as with previous technology waves, predicting winners is nearly impossible. Many current breakthroughs will become commodities in the next 5–10 years.
Having spent the last decade in the enterprise SaaS space, I find myself approaching AI with the curiosity of a child—eager to understand where enterprise AI is headed, which companies will emerge as leaders, and what opportunities lie ahead.
Enterprise AI: The role of Context
One of my core beliefs: ‘Context’ will be the real moat in the enterprise AI space.
Let’s define “context” for the purpose of this article. Context refers to industry/company/ or situation-specific information that can be fed into an LLM to enhance decision-making and generate more relevant, informed responses for the Enterprise workflows.
It is not just about intelligence; it is about getting the right information into the model at the right time. Companies that master context—by structuring, integrating, and feeding the most relevant data into AI models—will have a significant competitive edge.
The Hiring Analogy: Context in Decision-Making
My belief emerges from how we treat human intelligence while making hiring decisions. Think about hiring. For senior roles, companies prioritize industry experience(i.e. context of that industry) over raw intelligence. Why? Because experience provides context—enabling candidates to make informed decisions and navigate complex situations.
Junior roles, on the other hand, often emphasize raw intelligence, adaptability and learning potential, with the expectation that context will develop over time.
This analogy applies (should apply) to AI:
- General intelligence is valuable, but without context, AI models (will) produce generic or incomplete outputs (Generic LLMs will be/are good science/side projects but they will not produce enterprise grade results)
- Providing relevant, structured context (i.e. relevant data) improves AI performance, making outputs more accurate and actionable.
Where are we headed in Enterprise AI?
All my thinking is based on the below principles:
1. Convergence of AI Models
Performance across major foundational models will eventually converge. Today’s best model may not hold its lead for long. Once foundational model quality reaches a certain threshold, data context will become the primary differentiator.
2. Speed of Data Capture Will Be Key
Companies that build real-time data pipelines to continuously feed AI models with fresh, relevant, and proprietary data will gain a lasting advantage.
3. Integration of Enterprise Data Sources
Enterprise AI success will depend on how well businesses:
- Unify disparate data sources (ERP, CRM, internal documents, external data feeds).
- Apply the right permission structures to control data access.
- Feed structured, domain-specific data into AI models.
The real differentiator will not just be the model—it will be how companies manage, structure, and input proprietary data to create context-rich intelligence.
A strategic mistake: Waiting for the next model
Too many businesses are waiting for better AI models instead of optimizing how they feed data into existing models.
Success in enterprise AI will be determined by:
- The quality of the data being fed into the model.
- How well AI models are fine-tuned with real-time, proprietary context.
Companies should stop fixating on incremental model improvements and instead prioritize building a robust data strategy. History shows that transformative technologies—whether databases, CI/CD pipelines, cloud computing, or mobile—evolved over time. The real advantage went to companies that strategically positioned themselves to leverage these shifts, not those waiting for the technology to mature.
Opportunities in Enterprise AI (Because who doesn’t love capitalizing on major tech shifts?)
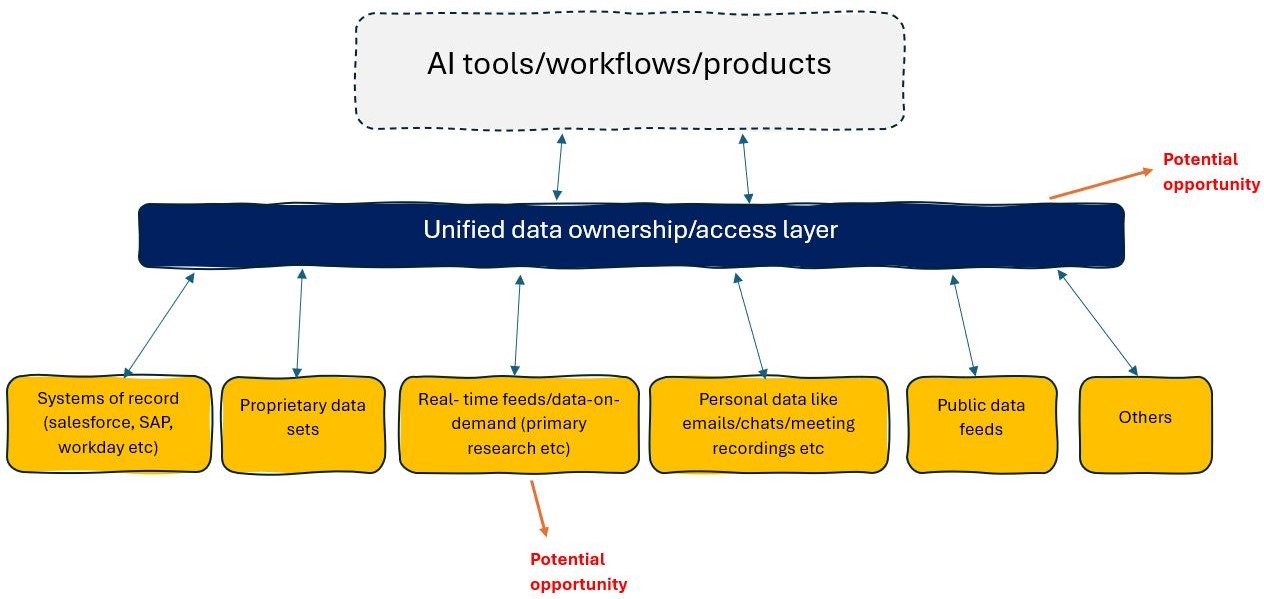
A key opportunity lies in building a unified data ownership and access layer on top of existing enterprise data. Additionally, developing frameworks and workflows that enable AI to access real-time, on-demand data (akin to primary research) presents another promising avenue for new entrants in the AI space.
1. Building a Unified Data Ownership Layer
A significant opportunity in enterprise AI lies in creating a software layer that classifies and governs data ownership and access across an organization. This layer would address key questions such as:
- Which data can be used for model training?
- Which data should AI have access to for answering queries?
- How should data access be structured based on user roles and permissions?
Enterprises rely on a complex web of tools and systems, making it impractical to replace everything overnight. Instead, an abstraction layer that integrates existing systems while ensuring structured, context-rich access to data could be a game-changer. This layer can not be static, this layer has to learn and evolve (e.g. a slack message from the CEO might be a good data point with a lot of weight but a financial report from the CFO holds more value. Also, which data sources to use in which types of workflows will be interesting)
I often hear the argument that traditional systems of record (SAP, Salesforce, etc.) will lose relevance as companies shift to AI-first workflows. While the business layer will undoubtedly evolve, assuming that enterprises can simply shut down their systems of record overnight is unrealistic. These platforms will adapt, enhancing their AI capabilities rather than being replaced outright.
The real opportunity for new entrants lies in unifying and enriching data across these systems of record—transforming raw enterprise data into structured, AI-ready context. This means determining which data is relevant for legal, operations, management, HR, and other workflows, ensuring AI can make informed, business-critical decisions.
Companies like Glean and Dropbox are already making intersting products in this space, but there is still a significant white space for innovation (more on this in another post).
2. Real-Time Data Feeds for AI Models/Data-on-Demand
Most AI models are trained on historical data, meaning their outputs are inherently backward-looking.
- New information is being generated at lightning speed.
- AI models need real-time primary research, external news feeds, and market intelligence to stay relevant.
Companies that can build pipelines for AI to request and ingest fresh data on demand will hold a competitive advantage. Companes in primary research space who can build this capability will benefit a lot.
Proprietary data: The ultimate AI Moat
In enterprise AI, proprietary data will be king. The companies that own exclusive, high-value datasets will dominate.
- Salesforce, HubSpot, and Zendesk have rich customer interaction data.
- SAP, Oracle, and Workday aggregate operational and financial data.
- Market research firms and survey platforms generate proprietary insights AI models cannot access elsewhere.
Enterprise software companies will not disappear. Just like the slow migration from on-prem to cloud, companies will not suddenly abandon Salesforce, ERP systems, or CRM tools. These platforms run core business operations—and they own the data that will fuel enterprise AI.
The Future of Enterprise AI: A Moving Moat
The real competitive advantage won’t be the AI model itself—it will be the ability to integrate dynamic, real-time context into AI models
Winning companies will:
✅ Own the most proprietary data (i.e. industry/problem specific context) or will have the ability to get the latest data at the fastest speed.
✅ Enable AI models to request and access the right data at the right time.
✅ Deliver real-time, actionable insights rather than static, generalized outputs.
AI models will improve over time, but the true moat—the advantage that compounds—will be data context. The companies that master it will win the enterprise AI race.
Disclaimer: https://vinaysachdeva.com/disclaimer/. The opinions expressed in the blog post are my own and do not reflect the view(s) of my employer.